Projects & Innovation
Innovation at our Core
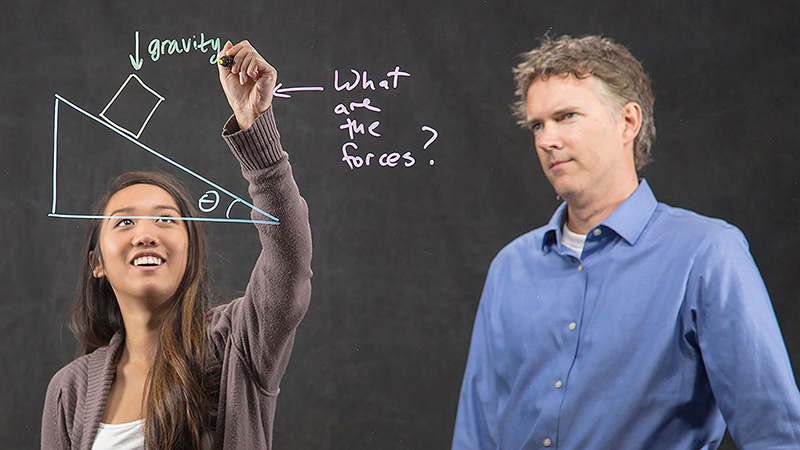
SDSU physics professor Matt Anderson, together with a team of ITS engineers are defying instructional norms with the introduction of the Learning Glass — an LED-lit glass board that faculty can write on while maintaining eye contact with their students.
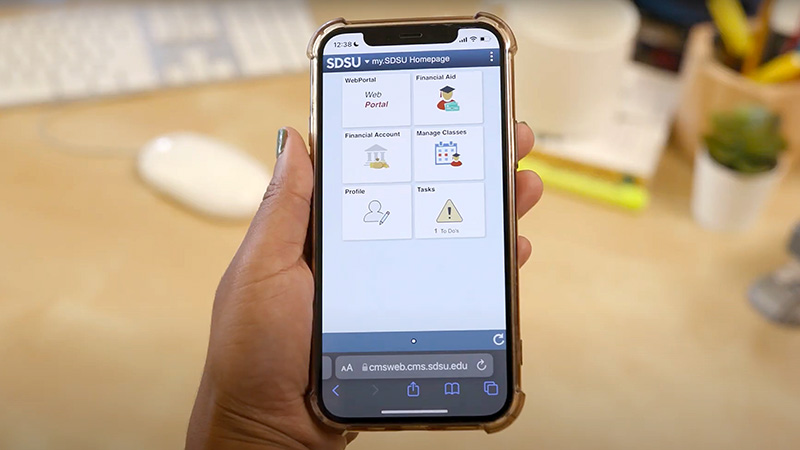
The my.SDSU project extends the student experience, encompassing faculty and staff support, data-driven strategies, and university investments. These elements enhance programs and services from admission to graduation, ensuring student success.
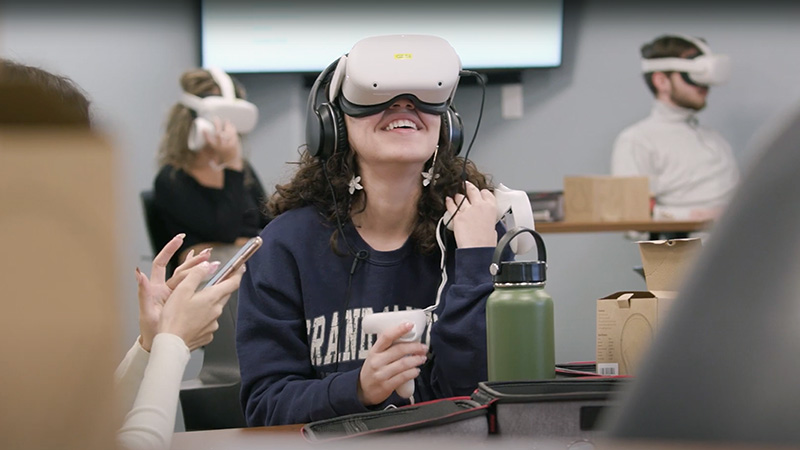
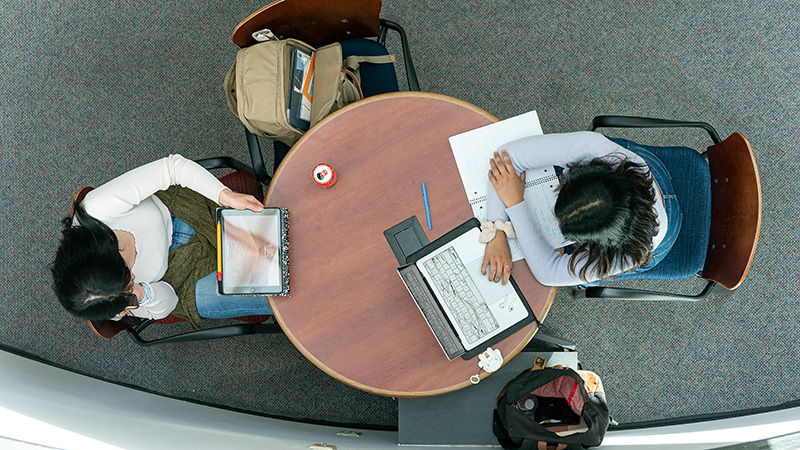
A New Kind of Hispanic-Serving Institution
SDSU will forge a path to become an R1 Doctoral University where excellence and access converge.
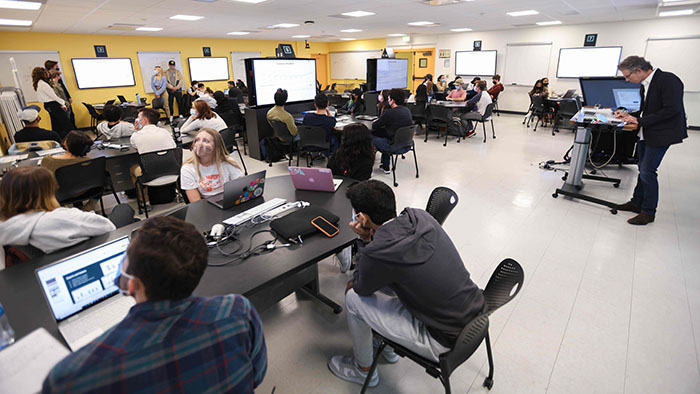
Learning Research Studios
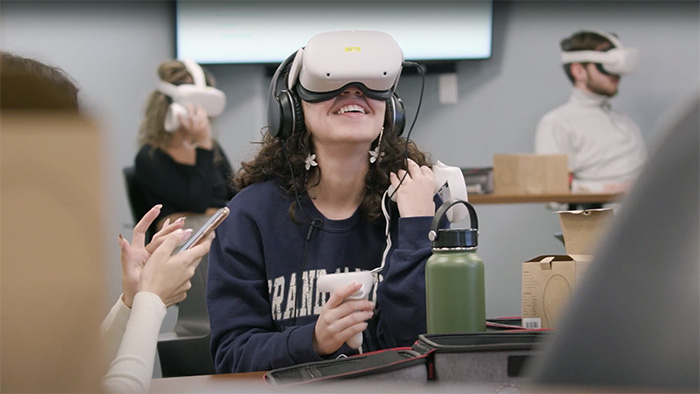
Virtual Immersive Teaching and Learning (VITaL)
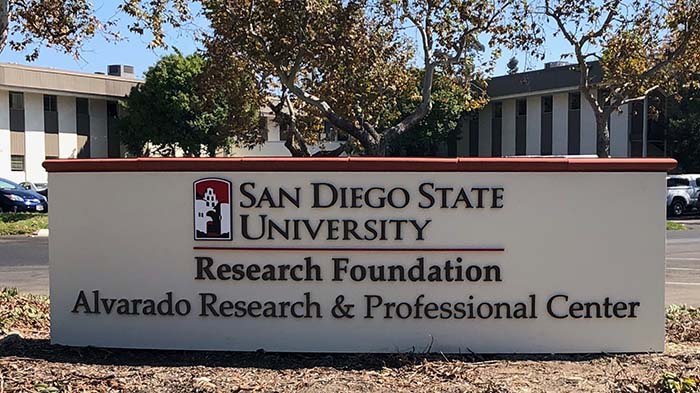
Alvarado Research Park Infrastructure
Equity & Inclusion
SDSU will be a global leader in advancing diversity, equity, and inclusion in research, teaching and in community engagement.
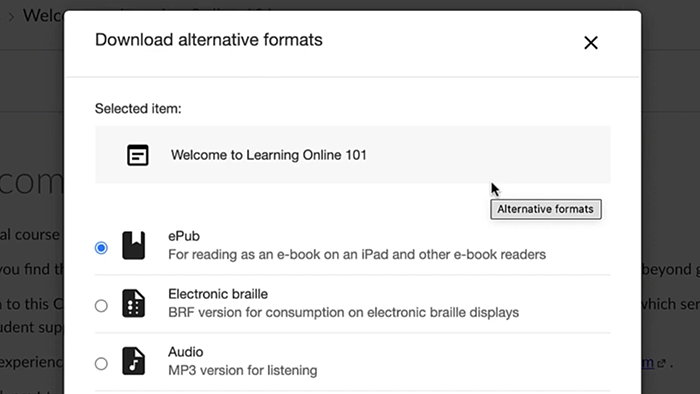
Accessibility
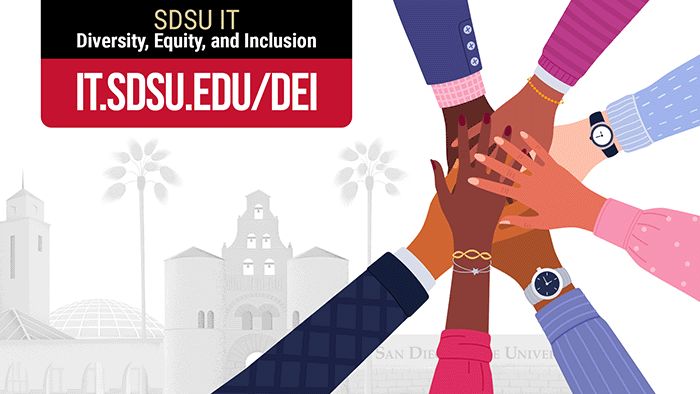
Diversity, Equity & Inclusion
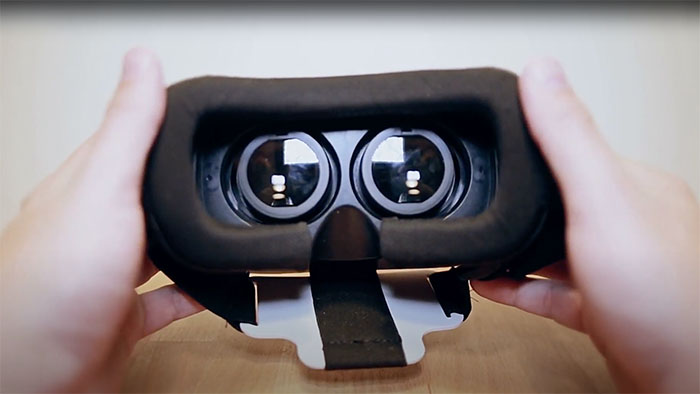
Empathy Lens
Resilience, Designed to Thrive
SDSU is committed to building a resilient and sustainable university through innovative practices.
Students at Our Core
SDSU commits to a future where all students are able to achieve their greatest potential.
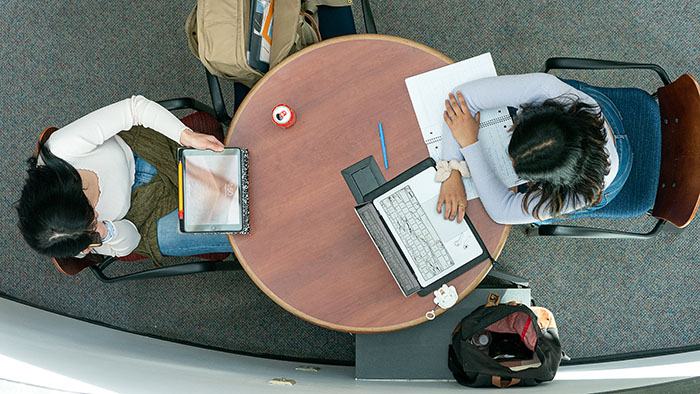
Affordable Learning Solutions
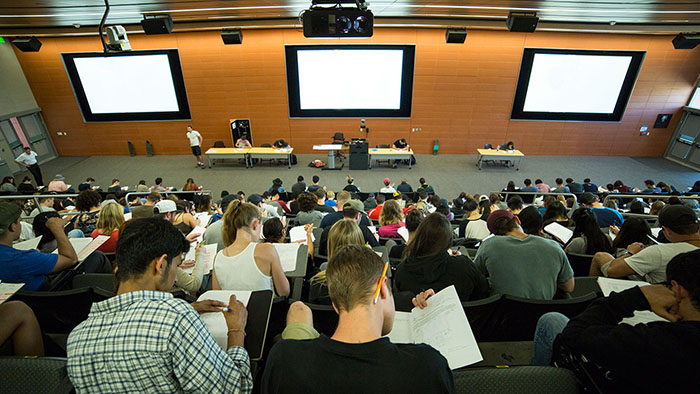
Enhanced Learning Environments
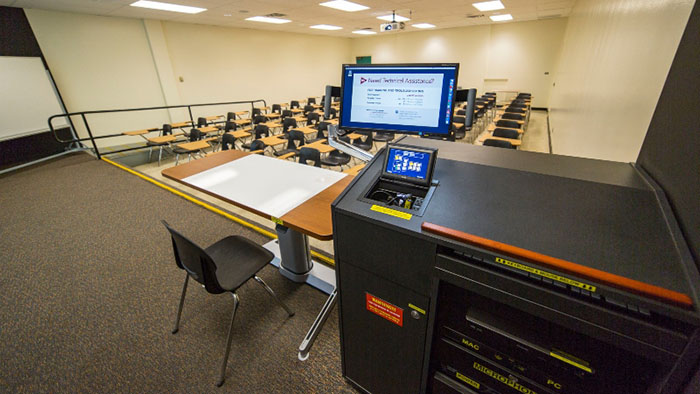
Learning Space Rating System
We Are SDSU
SDSU will expand its global impact, unifying the university through a common mission and identity.
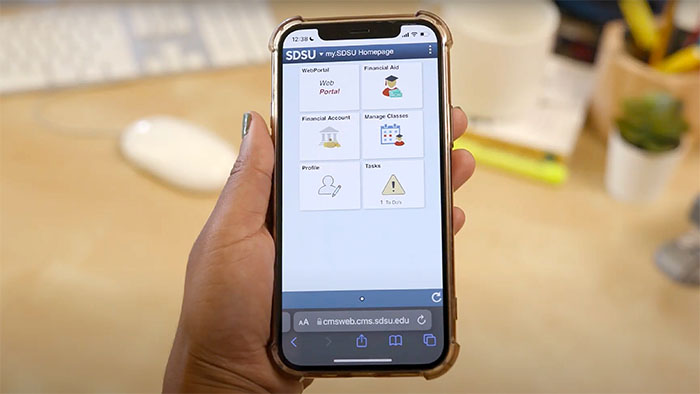
my.SDSU
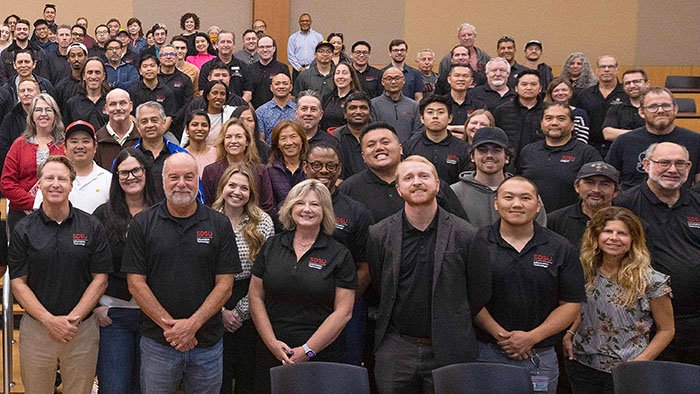
OneIT Community
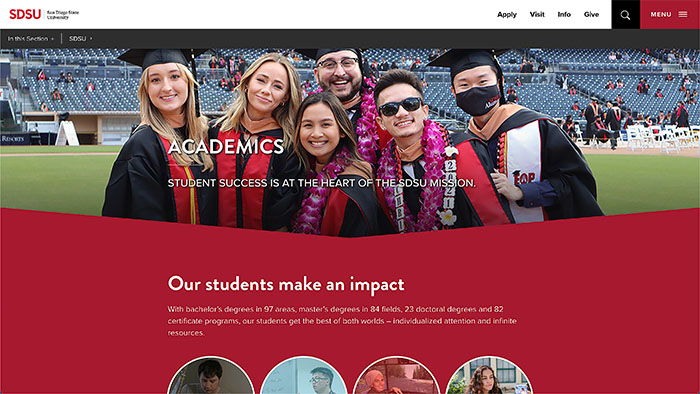